A brain-computer interface that records signals in the motor cortex can synthesize speech from activity in a user’s brain
Two years ago, a 64-year-old man paralyzed by a spinal cord injury set a record when he used a brain-computer interface (BCI) to type at a speed of eight words per minute.
Today, in the journal Nature, scientists at the University of California, San Francisco, present a new type of BCI, powered by neural networks, that might enable individuals with paralysis or stroke to communicate at the speed of natural speech—an average of 150 words per minute.
The technology works via a unique two-step process: First, it translates brain signals into movements of the vocal tract, including the jaw, larynx, lips, and tongue. Second, it synthesizes those movements into speech. The system, which requires a palm-size array of electrodes to be placed directly on the brain, provides a proof of concept that it is possible to reconstruct natural speech from brain activity, the authors say.
brain signals into movements of the vocal tract, including the jaw, larynx, lips, and tongue. Second, it synthesizes those movements into speech. The system, which requires a palm-size array of electrodes to be placed directly on the brain, provides a proof of concept that it is possible to reconstruct natural speech from brain activity, the authors say.
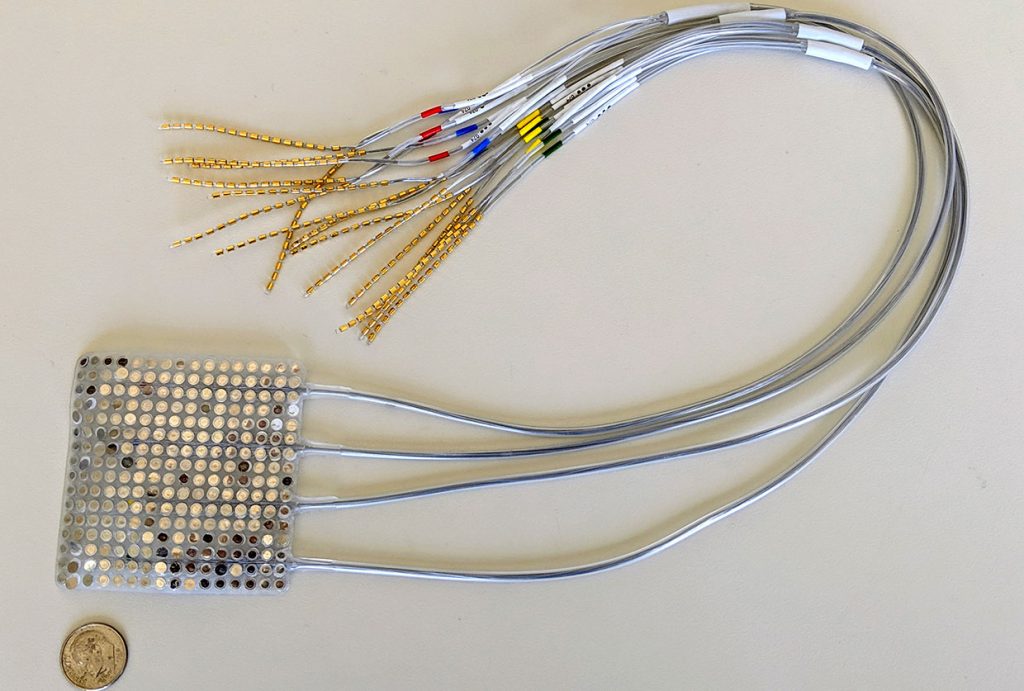
Researchers at the University of California, San Francisco, used an array of intracranial electrodes similar to this one to record participants’ brain activity for a new study.
Many studies focus on decoding sounds or whole words from brain activity, but decoding thoughts alone is “very difficult,” said study leader and UCSF neurosurgeon Edward Chang at a press briefing on the study. “We are explicitly trying to decode movements in order to create sounds, as opposed to directly decoding sounds.”
“It’s a beautifully designed, well-executed study of how to directly decode speech from brain signals,” says Marc Slutzky, head of a neuroprosthetics laboratory at Northwestern University, who was not involved in the research.
Yet translating the technology to clinical practice will be a challenge, Slutzky adds: “There are currently no FDA-approved devices using the types of electrodes they used in high-channel capacity (they used 256 channels here), so that remains a hurdle. But I believe this will eventually be overcome.”
Chang’s paper is the most recent in a string of efforts applying neural networks—a set of algorithms loosely modeled after the human brain and often applied to deep learning—to interpret sound from brain activity. Last week, two independent teams—Slutzky’s lab at Northwestern and Nima Mesgarani’s lab at Columbia University—each published papers, in the Journal of Neural Engineering and Scientific Reports, respectively, using neural networks to reconstruct speech from brain activity in the sensory network. The current study differs from these studies by analyzing brain activity in the motor cortex instead.
“What approach will ultimately prove better for decoding the imagined speech condition remains to be seen, but it is likely that a hybrid of the two may be the best,” Mesgarani told IEEE Spectrum.
Each of the recent studies, Chang’s included, relies on electrodes placed directly in or on the brain via surgery. Though Facebook has claimed it is developing a noninvasive technology able to read out 100 words per minute from user’s brains, it hasn’t backed up that claim. External electrodes simply cannot provide precise enough data from small brain regions, experts agree. The BrainGate consortium, which published the study with the eight-words-per-minute record in 2017 and a recent paper of BCI users controlling off-the-shelf tablets, also relies on implanted brain chips.
In the current study, Chang and colleagues gathered data from an array of electrodes implanted onto the speech motor cortex of five patients undergoing epilepsy treatment. The researchers recorded brain signals as the patients spoke several hundred sentences aloud. The sentences, including “Is this seesaw safe?” and “At twilight on the twelfth day we’ll have Chablis,” were specifically chosen to include all the phonetic sounds of the English language.
Next, the researchers employed a neural network to decode those high-resolution brain signals into representations of the movements of the vocal tract—essentially transforming brainwaves into a model of physical motions that would produce sound, such as the movements of lips, tongue, or jaw. This work was based on a model the team published last year in the journal Neuron.
Finally, they used a second neural network to synthesize those digital representations into an audio signal, and asked volunteers to listen to it. In a trial of 101 sentences, listeners could identify and transcribe the synthesized speech fairly well with the help of a word bank: Listeners perfectly transcribed 43 percent of sentences with a 25-word vocabulary pool and 21 percent of sentences with a 50-word pool. Overall, about 70 percent of words were correctly transcribed. Next steps in the research include making the audio tract more natural and intelligible, said Chang.
In an intriguing addition to the study, one participant was asked to speak the sentences without any sound, miming the words with his vocal tract. The BCI was able to synthesize intelligible speech from the miming of a sentence, suggesting the system can be applied to people who cannot speak.
The decoded movements of the vocal tract were similar from person to person, suggesting it is possible to create a sort-of “universal” decoder to share among individuals. “An artificial vocal tract modeled on one person’s voice can be adapted to synthesize speech from another person’s brain activity,” said Chang.
One major limitation of the study was that it engaged only participants without speech disabilities. In the future, the team hopes to conduct clinical trials testing the technology on patients who cannot speak, said Chang.
Source: www.spectrum.ieee.org