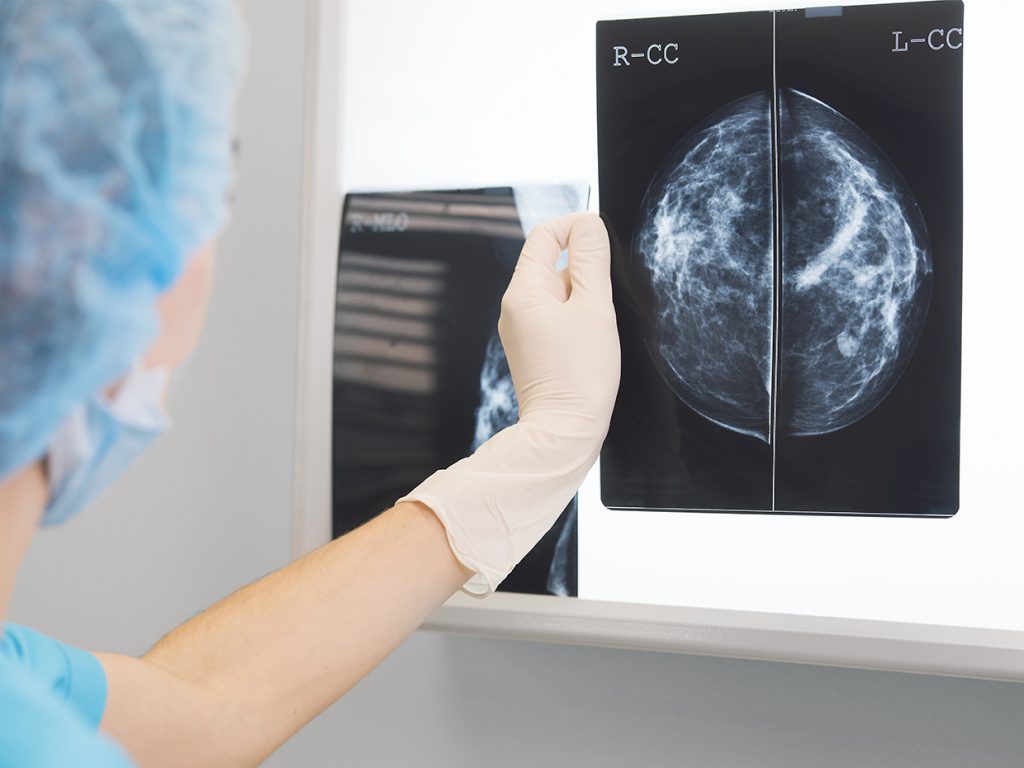
Photo: iStockphoto
Women with dense breasts have a greater risk of undergoing mammogram screenings that miss signs of breast cancer. That’s why 30 U.S. states legally require that women receive some notification about their breast density. A new study suggests that commercial software for automatically classifying breast density can perform on par with human radiologists: a finding that could encourage wider use of automated breast density assessments.
Increased breast density represents “one of the strongest risk factors for breast cancer,” because it makes it more difficult to detect the disease in its early stages, explained Karla Kerlikowske, a physician and breast cancer researcher at the University of California, San Francisco. Dense breast tissue may also carry a higher risk of developing breast cancer.
Breast density refers to the proportion of “nondense” fatty tissue to other “dense” tissue, containing milk ducts and glands, within the breast. For women with dense breasts, physicians may recommend supplemental screening or changes to screening frequency in order to detect breast cancer earlier.
The new study suggests automated screenings are just as accurate as doctors in determining breast density from a mammogram, and may have other advantages as well. In addition to comparing assessments of breast density, the study funded by the National Cancer Institute also compared the automated and human breast density assessments on two measures related to their ability to predict a woman’s risk of developing breast cancer.
First, the study looked at how well the software and clinical assessments by radiologists predicted breast cancer risk through mammography screening. Second, it considered how well they predicted the risk of “interval invasive cancer” that is not caught by mammography screening and is instead diagnosed through direct clinical examination. In both cases, the software assessments compared well with radiologists’ assessments in predicting those cancer risks.
“Automated density measures are more reproducible across radiologists and facilities,” said Kerlikowske. “Using automated measures will allow accurate identification of women who have dense breasts and are at high risk of an interval cancer so these women can have appropriate discussions of whether supplemental imaging is right for them.”
To compare automated and human assessments, Kerlikowske and her colleagues combined data from two case-control studies based on the breast imaging databases of the San Francisco Mammography Registry and the Mayo Clinic. Their results are published in the 30 April 2018 online issue of the journal Annals of Internal Medicine.
Radiologists estimate the percentage of dense breast tissue based on a subjective visual examination of mammogram images. They categorize the breast tissue under four classes defined by the Breast Imaging Reporting and Data System (BI-RADS): (a) almost entirely fatty, (b) scattered fibroglandular densities, (c) heterogeneously dense, and (d) extremely dense.
But subjective assessments by radiologists can lead to inconsistencies. Previous research has found that 10 percent of women received a different breast density assessment when examined by the same radiologist in consecutive mammograms. That rises to 17 percent when their mammography images are examined by two different radiologists.
Commercial software based on machine learning algorithms offers the promise of providing a more reliable and consistent measure of breast density that is not dependent upon an individual radiologist’s judgment. One example is a program called Volpara that can estimate dense or nondense tissue volume in each pixel of mammogram images. Its algorithms use that as the basis for calculating overall breast thickness and dense tissue volume in each breast.
Volpara represents one of the more popular examples of such software, given that it currently covers about 3.2 percent of U.S. women and is undergoing trials in Europe. For that reason, the new breast density study focused on comparing Volpara’s performance with the performance of radiologists. But researchers may want to perform additional comparative studies for other software.
Another lingering question is how cost-effective the automated approach would be compared with human radiologists. That would require looking at the cost of a radiologist’s time to read and record breast density on mammograms for a year versus the cost of using software, Kerlikowske said. Anecdotally, one radiologist told her that he estimated the software might save him an hour a day.
The questions of cost and overall effectiveness also appear in an editorial published in the same journal issue as the new study. Written by Joann Elmore, a physician at the University of California, Los Angeles, and Jill Wruble, a radiologist at the Yale School of Medicine in New Haven, the editorial points to the use of another technology, computer-aided detection (CAD) for highlighting abnormal areas in mammography images, as a cautionary tale for using automated tools in breast cancer screening.
Elmore and Wruble noted that CAD’s value has been questioned despite the fact that it has become widely used at a cost of more than $400 million per year. They cite studies suggesting that CAD’s use either provides no improvement in detecting breast cancer or performs with worse accuracy in comparison with the scrutiny of human radiologists.
“Like CAD, automated density measurement has the potential to improve reproducibility and workflow efficiency,” Elmore and Wruble write. “However, we are in an era of ‘choosing wisely’ and seeking value in health care. Therefore, we must be cautious before implementing and paying for medical technology.”
For now, Kerlikowske and her research team are running additional studies to explore how machine learning software—particularly software based on deep learning algorithms—can help physicians identify women who many need additional imaging beyond mammograms to reduce their breast cancer risk.
source: www.spectrum.ieee.org